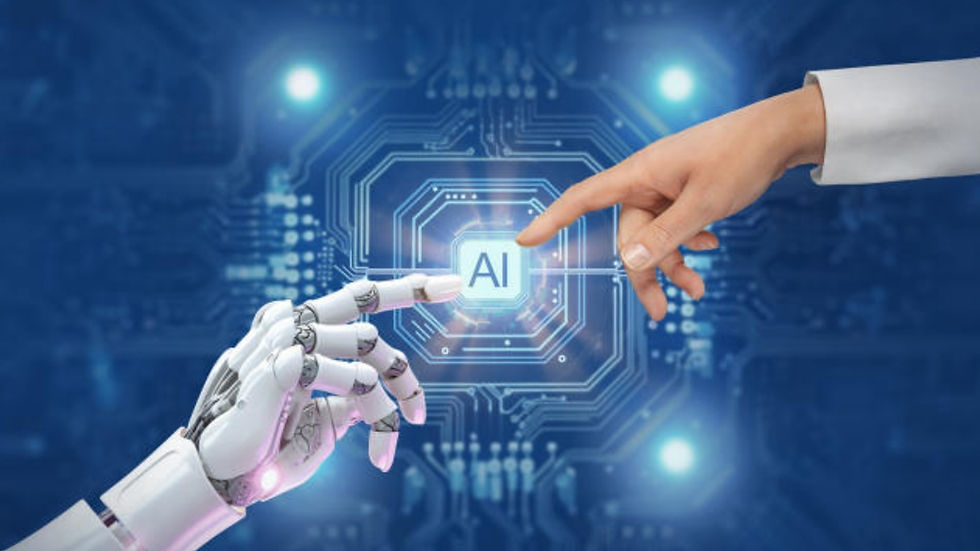
Generative AI, despite its impressive capabilities, has a fundamental flaw: it often fabricates information, presenting fiction as fact with unwarranted confidence. This issue isn't new. When ChatGPT launched in 2022, it quickly became apparent that generative AI struggles to distinguish between fact and fiction. Despite this, the tech industry continues to integrate AI deeply into the digital ecosystem, seemingly overlooking these inherent flaws.
Generative AI's tendency to produce inaccurate information may seem harmless when it comes to recipes or video suggestions. However, this unreliability becomes a significant concern in critical fields like medicine, finance, and law, where precision is paramount, and errors can have serious consequences.
Recent investigations have highlighted the ongoing issues with AI accuracy. Perplexity, a popular AI-powered "answer engine," has been criticized for delivering inaccurate and plagiarized responses. Wired even dubbed it a "bulls--t machine," echoing frustrations expressed by many users with Google's AI search summaries.
Generative AI tools, such as ChatGPT and Dall-E, initially wowed users with their ability to mimic famous authors and artists. These tools are now being marketed as comprehensive work, knowledge, and interaction solutions. However, their core strength lies more in brainstorming and generating ideas than in providing reliable answers.
AI's fabrications, often called "hallucinations" or "confabulations," are not random bugs but intrinsic to how these systems function. Large language models, like Google's Gemini and OpenAI's GPT, predict the next word in a sequence without actual understanding, leading to inconsistent and sometimes erroneous outputs. Users expect AI to behave with the consistency and logic of traditional computing tools. However, generative AI incorporates an element of randomness, leading to unpredictable results. This fundamental discrepancy between user expectations and AI capabilities is a significant challenge.
Potential Solutions
The tech industry has several paths to address this problem:
1. Enhancing Accuracy: Improving data quality and training processes to make AI more reliable. However, achieving perfect accuracy is complex, and less aggressive AI might seem less useful.
2. Reducing Confidence: Training AI to express uncertainty and admit when it doesn’t know an answer. This approach requires AI to recognize its limitations, which is challenging to implement.
3. Reframing the Narrative: Accepting AI's tendency to fabricate as a creative feature rather than a flaw, positioning these tools as aids for brainstorming rather than sources of factual information. This would likely result in a smaller market but could set realistic user expectations.
Selling generative AI as a groundbreaking technology becomes challenging when its outputs are unpredictable and sometimes incorrect. Silicon Valley faces the difficult task of managing these expectations while striving for technological advancements. AI developers must navigate these complexities to balance innovation with reliability.